The Astounding Power of Large Language Models (LLMs) in 2023
Read this blog article to discover how Large Language Models (LLMs) are transforming the world of artificial intelligence. These cutting-edge systems are designed to process and generate human-like text, opening up new possibilities for language understanding and generation.
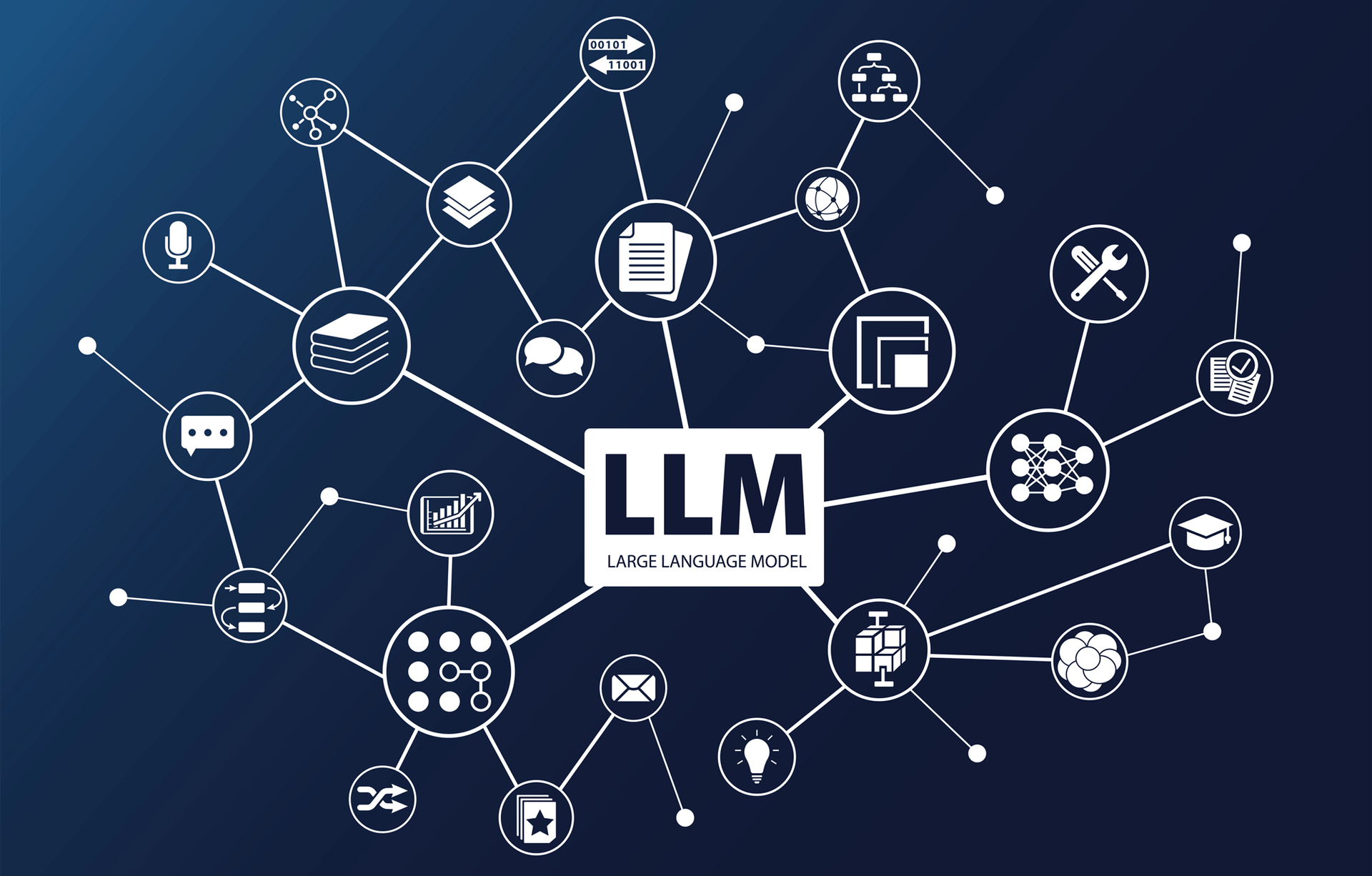
Discover the awe-inspiring realm of large language models, powerful AI systems meticulously honed to understand and mimic human-like text. These models, fueled by neural networks inspired by the human brain, excel at unraveling language patterns.
What sets these language models apart is their sheer magnitude, measured by the number of parameters they possess. With millions to billions of parameters at their disposal, these models masterfully capture the intricacies and nuances of language, enabling them to make informed decisions and generate text with remarkable finesse.
Creating and training these behemoth models is a Herculean task, demanding colossal computational power and vast amounts of data. For instance, OpenAI's GPT-4 (the fourth iteration of "Generative Pre-trained Transformers") boasts a staggering 1.7 trillion parameters—an unrivaled titan in the language model realm.
Building models on such a grand scale often entails fine-tuning. Initially, these models are pre-trained on vast swaths of internet text, immersing themselves in different words, phrases, and sentence structures. Following this pre-training phase, specific datasets finely tune the models to suit particular tasks, like translation or text completion. This process optimizes performance and tailors the model to specific applications.
Beyond their gargantuan size, large language models also benefit from human guidance. Incorporating feedback from human reviewers further elevates these models by imbuing them with accuracy, coherence, and alignment with human values. The continuous feedback cycle refines and enhances these AI systems.
The capacities of large language models extend far and wide, showcasing their prowess in domains like question answering, article writing, translations, and even the generation of creative content like poems and stories. By intelligently processing and generating text on an unprecedented scale, these models possess the potential to empower humans across diverse tasks.
Naturally, challenges accompany these masterpieces. The dense, parameter-heavy nature of these models demands immense computational resources for optimal training and operation. The colossal amount of computations, fondly referred to as flops, that large models require strains hardware capabilities. Researchers and engineers persevere, constantly fine-tuning and optimizing these models to strike a balance between efficiency and performance.
Reliability and ethical application of these models rely upon robust evaluation datasets. Adversarial evaluation datasets push these models to their limits, presenting complex examples and potential biases. This allows researchers to uncover any errors or biases, paving the way for improvements and ensuring fairness and reliability in the analysis and generation of text.
In conclusion, these monumental language models, birthed from extensive training and vast parameters, hold the potential to revolutionize human interaction with technology. As tireless researchers and engineers continue to push the boundaries of AI, the development and refinement of large language models promise thrilling advancements in natural language understanding and generation. Brace yourself for a new era of AI breakthroughs.
What are Large Language Models?
The Astounding Benefits of LLMs
Revolutionalizing AI: The Power of LLMs
The world of artificial intelligence has seen a remarkable transformation thanks to the rapid growth of language models. These models, powered by neural networks, have become more advanced and sophisticated, pushing the boundaries of what AI can accomplish in understanding and generating human language.
One key measure of a language model's capacity is its parameter count, representing the number of learnable parameters. The larger the model, with millions or even billions of parameters, the better it can process and comprehend text. These large-scale models have emerged as the cutting-edge in AI research and development.
So, what's driving the attention on these massive language models? Larger models excel at capturing complex patterns and dependencies in data. With their extensive parameter space, they can learn intricate linguistic patterns, including grammar, syntax, and semantics. This remarkable capability enables them to generate coherent and contextually relevant text, making them invaluable for language-related tasks. And as the model size increases, so does its contextual understanding.
Neural language models, such as OpenAI's GPT-4, are based on transformer architectures that excel at learning the relationships between words in context. By considering the surrounding context, these models can accurately decipher the meaning of individual words, leading to precise and meaningful predictions. This contextual understanding is key to producing text that is not only grammatically correct but also contextually coherent.
Another advantage of these large models is their pre-training on massive datasets from the internet. Before being fine-tuned for specific tasks, these models undergo extensive pre-training on vast amounts of text data, which gives them a broad knowledge base across various topics and domains. Leveraging these pre-trained models saves time and resources that would be spent on training from scratch, accelerating AI development.
Despite their advantages, large-scale language models also come with challenges. Their size requires significant computational power and time, making training and inference expensive and time-consuming. Meeting these computational demands necessitates advanced hardware infrastructure and efficient parallel processing techniques.
Data requirements are another hurdle researchers and developers face. These models heavily rely on labeled training data for optimal performance, but gathering and curating extensive datasets is a daunting and resource-intensive task. Additionally, ensuring the training data represents a wide range of linguistic patterns, styles, and contexts adds complexity to the training process.
Ethical concerns surrounding large-scale language models are essential to address. These models learn from internet text, which may contain biases and offensive content. Responsible use and monitoring are crucial to prevent the propagation and amplification of harmful information. Implementing mechanisms to detect and mitigate biases ensures the generation of fair, unbiased, and inclusive content.
Interpretability is also a challenge with large neural networks. Understanding how these models work and make decisions is difficult, hindering troubleshooting and explaining model predictions. Establishing techniques to interpret and explain their behavior is vital for responsible and reliable use.
In conclusion, large-scale language models have revolutionized natural language processing and understanding. Their ability to capture complex patterns, comprehend context, and possess vast knowledge makes them incredible tools for language-related applications. However, addressing challenges such as computational requirements, data availability, ethical concerns, and interpretability is crucial for advancing these AI language models responsibly.
Unleashing the Power of Neural Networks
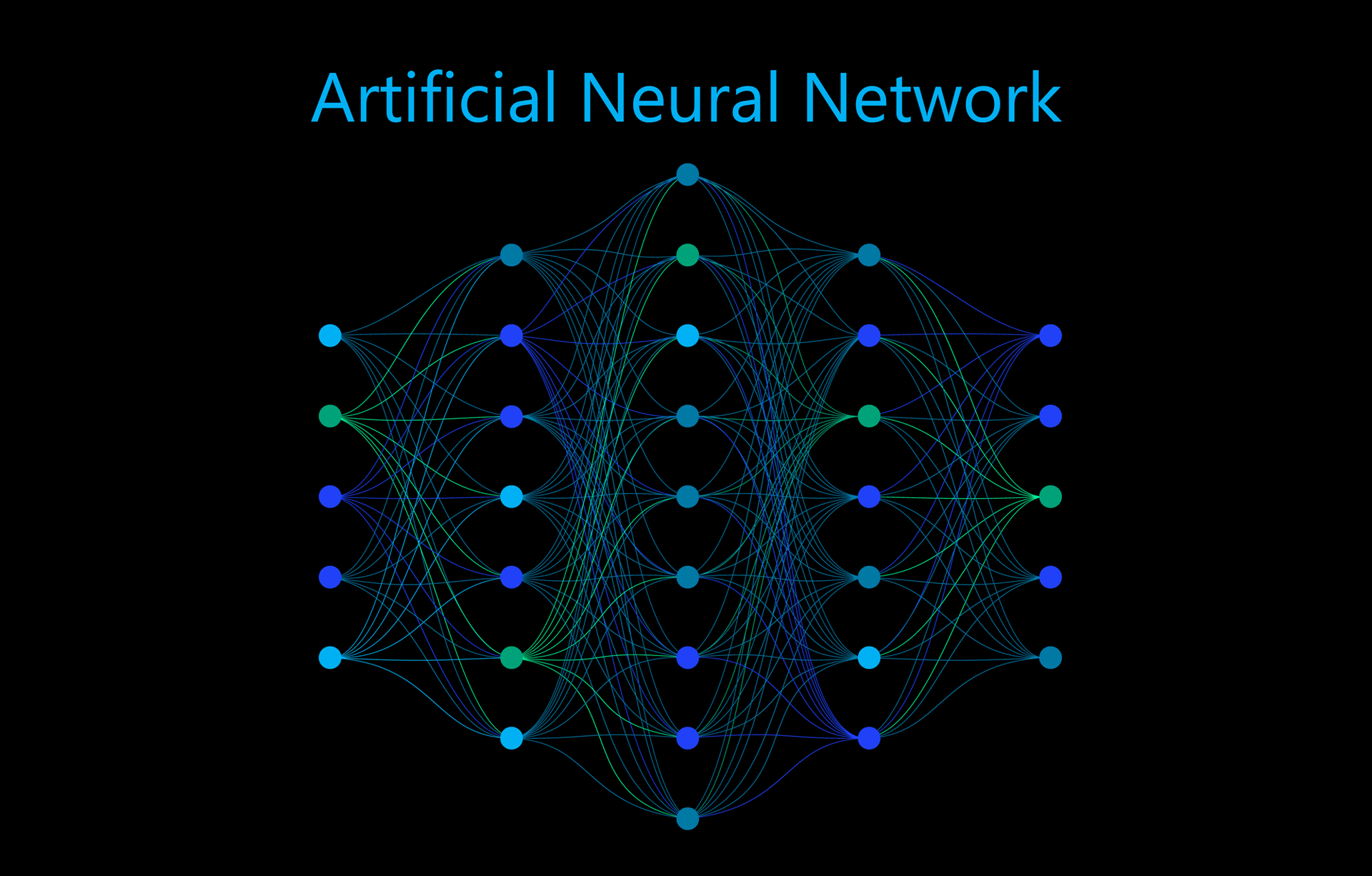
Discover how neural networks have revolutionized the world of artificial intelligence and machine learning. These advanced algorithms, inspired by the human brain, are capable of processing complex patterns and making intelligent predictions.
Imagine a network of interconnected "neurons" working together to analyze and process data. Each neuron takes input, performs calculations, and passes its output to the next layer of neurons. This intricate web of connections allows neural networks to learn and adapt, making them highly versatile and efficient in various tasks.
One of the biggest advantages of neural networks is their ability to learn from data. Through a process called backpropagation, the network adjusts its neurons' weights and biases to minimize the difference between predicted and actual outcomes. This enables neural networks to identify patterns, make predictions, and accurately classify data.
Neural networks have proven their prowess in numerous domains, from image recognition and natural language processing to speech recognition and recommendation systems. In image recognition, they can analyze pixels and identify objects, powering applications like facial recognition and self-driving cars. In natural language processing, they can understand and generate human-like text, enabling virtual assistants and chatbots to engage in meaningful conversations.
The evolution of neural networks has been propelled by larger datasets and more powerful hardware. Deep learning, a subfield of neural networks, introduces networks with multiple hidden layers. These deep neural networks have achieved remarkable accuracy in complex tasks, often surpassing human-level performance.
Despite their incredible capabilities, neural networks do face challenges. They require significant computational resources and labeled data for training. Overfitting, where the network becomes overly dependent on the training data, and interpretability, understanding the reasoning behind predictions, are areas of ongoing research.
Neural networks continue to push the boundaries of artificial intelligence, allowing machines to process information, learn, and make decisions in unimaginable ways. As researchers and engineers refine these models, we can expect neural networks to play an increasingly pivotal role in shaping the future of technology.
Neural Networks in Language Models
Discover how neural networks have transformed language models, propelling us into a new era of understanding and generating human language. Language models, statistical models that capture the intricate patterns and structure of natural languages, now have unprecedented capabilities thanks to neural networks.
Neural networks possess the unique ability to learn from vast amounts of data, allowing them to grasp the nuances of grammar, syntax, and semantics. This enables them to accurately predict the next word in a sentence, complete sentences, and even produce text that is indistinguishable from human writing.
One standout example of a neural language model is GPT-4, developed by OpenAI. Boasting an astounding 1.7 trillion parameters, GPT-4 has been trained on an extensive corpus of internet text, making it a highly knowledgeable resource on numerous topics.
Neural language models rely on transformer architectures to handle sequential data, such as sentences, by understanding the contextual relationships between words. This allows the models to generate text that is both coherent and meaningful.
The training process for neural language models involves exposing the model to vast amounts of text and fine-tuning its parameters to achieve a specific objective. Through careful refinement, these models can generate high-quality text tailored to specific styles or tones.
One prevailing challenge for neural language models is prompt engineering. Crafting effective instructions or queries is crucial to leveraging the model's capabilities and obtaining accurate and relevant results.
Human feedback is invaluable in training and enhancing neural language models. Through iterative refinement and exposure to correct examples, these models continuously improve and adapt.
While neural language models have demonstrated exceptional abilities, ethical concerns arise regarding potential biases and inappropriate content generation. Initiatives are underway to develop evaluation datasets and assessment methodologies to mitigate risks and ensure responsible use.
In conclusion, neural networks have revolutionized language models, pushing the boundaries of natural language understanding and generation. With their vast capacity for learning, models like GPT-4 have the potential to excel in language completion, translation, and creative writing. Yet, responsible harnessing of their power through prompt engineering, fine-tuning, and human feedback remains crucial.
Revolutionize Language Modeling with Neural Networks: Benefits and Challenges
Read below to discover the incredible advantages of using neural networks for language modelling and the potential pitfalls to watch out for.
Advantages:
Unlock Complex Patterns: Neural networks excel at unraveling intricate patterns and interdependencies in data. This makes them an ideal choice for language-related tasks, enabling them to grasp the complex linguistic patterns found in text data. Neural language models can comprehend grammar, syntax, and meaning, resulting in the generation of coherent and contextually relevant text.
Context is Key: Powered by transformer architectures like GPT-4, neural language models focus on understanding contextual relationships between words. This empowers the model to gauge the meaning of a word based on its surrounding context, leading to more accurate predictions. With contextual understanding, generating meaningful and coherent text becomes effortless.
Tap into Pre-trained Models: Neural language models are commonly pre-trained using massive datasets from the internet. This pre-training helps the model absorb knowledge about various topics and enhances its grasp of natural languages. Leveraging these pre-trained models saves developers valuable time and resources that would otherwise be spent on training from scratch.
Challenges:
Power-Hungry Processing: Neural language models with millions or even billions of parameters require substantial computational resources for training and inference. Training such large models can consume considerable time and computational power. Additionally, deploying these models for real-time applications may pose challenges due to their demanding computational requirements.
The Hunger for Data: Achieving optimal performance with neural networks for language modeling requires vast amounts of labeled training data. Acquiring and curating such datasets can be a time-consuming and resource-intensive task. Moreover, obtaining labeled data that covers a wide range of linguistic patterns and styles presents its own set of challenges.
Navigating Ethical Concerns: Large-scale neural language models raise concerns about the generation of biased or inappropriate content. These models learn from diverse data sources, including internet text, which may contain biases and offensive content. Ensuring responsible use and closely monitoring the output of these models is essential to prevent misuse or the spread of harmful information.
The Enigma of Interpretability: Neural networks are often referred to as "black boxes" due to their complex internal workings and decision-making processes. This lack of transparency poses challenges when troubleshooting, explaining model predictions, or addressing biases in the output. Developing techniques to interpret and explain the behavior of neural language models is crucial.
In conclusion, the advantages of neural networks in language modeling are undeniably remarkable, offering the ability to unravel complex patterns, understand context, and leverage pre-trained models. However, they also present challenges such as computational requirements, data dependencies, ethical concerns, and interpretability issues. Triumphing over these hurdles is crucial in harnessing the full potential of neural networks for language modeling while ensuring ethical and unbiased usage.
The Power of Megatron-Turing NLG and GPT-4
In the dynamic realm of artificial intelligence, language models have emerged as the forefront of remarkable advancements in AI research. Two standout models, Megatron-Turing NLG and OpenAI's GPT-4, have revolutionized AI language capabilities and set new benchmarks for the field.
Megatron-Turing NLG stands apart with its unrivaled size and parameter count. Developed at Stanford University, this model pushes the boundaries of AI with an astounding number of parameters. Its immense size enables precise and speedy processing and analysis of natural languages, making it an invaluable tool for a wide range of applications.
OpenAI's GPT-4 has mesmerized the AI community with its mind-boggling 1.7 trillion parameters. With this colossal capacity, GPT-4 showcases exceptional language generation abilities. It can produce coherent, contextually relevant text that is strikingly human-like, showcasing an in-depth understanding of grammar, syntax, and semantics. GPT-4's performance sets an entirely new standard in language modeling.
Megatron-Turing NLG and GPT-4 undergo rigorous training, starting with pre-training on massive datasets extracted from the internet. This phase allows the models to absorb vast knowledge and become proficient in various domains. Fine-tuning follows, where the models specialize in specific tasks, amplifying their language generation prowess even further.
Nevertheless, the use of these colossal AI language models comes with its own set of challenges. Their massive size demands substantial computational resources, making training and inference processes computationally costly and time-consuming. Implementing and maintaining the necessary infrastructure becomes a practical and cost-related hurdle, especially when deploying these models in real-time applications.
Another challenge lies in acquiring labelled training data. Megatron-Turing NLG and GPT-4 heavily rely on extensive datasets to achieve optimal performance. Compiling and curating such vast datasets requires considerable time, effort, and resources. Ensuring the representation of diverse linguistic patterns, styles, and contexts adds complexity to the training process.
Ethical concerns also arise when harnessing these large AI language models. The generation of biased or inappropriate content poses significant risks as the models learn from internet text, which may contain biases and offensive material. Mindful usage and vigilant monitoring are crucial to prevent the dissemination of harmful information. Implementing mechanisms to detect and mitigate biases ensures fair, unbiased, and inclusive content generation.
Furthermore, comprehending the inner workings of these enormous neural networks presents its own set of challenges. The complexity of these models hampers troubleshooting, explaining outputs, and effectively addressing biases. Ongoing research efforts to interpret and explain the behavior of these language models are pivotal in guaranteeing their responsible and dependable use across various applications.
Megatron-Turing NLG and GPT-4 exemplify the boundless possibilities and challenges of pushing AI language models to the next level. As these models continue to advance, they have the potential to revolutionize industries and empower AI-driven solutions. However, it is essential to navigate the challenges and ensure their ethical and responsible use to unlock their full potential for positive impact worldwide.
Pre-Training and Fine-Tuning Models
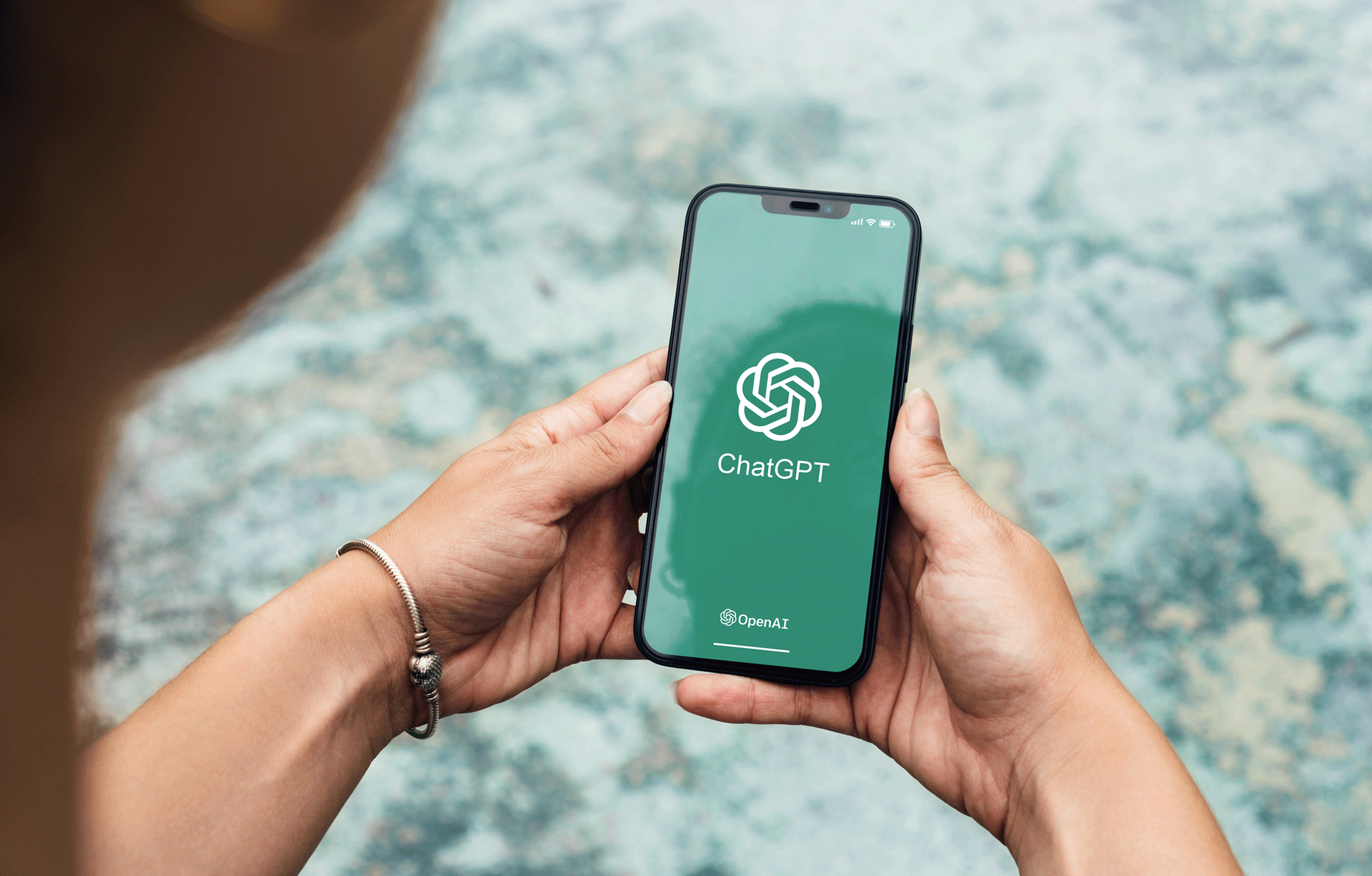
Pre-trained and fine-tuned AI models are changing the game by revolutionising natural language processing and understanding.
Pre-training, the initial phase, is where the magic begins. By exposing the model to massive amounts of unlabeled text data from the internet, it learns the statistical patterns and structures of natural language. Syntactical rules, grammar, and even semantics become second nature, resulting in contextually relevant and coherent text generation.
But that's not where it stops. Enter the fine-tuning process. The model is trained on labeled and task-specific datasets, tailor-made for specific applications and domains. This fine-tuning exercises its language generation capabilities, making it an invaluable tool for tasks like text generation, translation, summarization, and chatbots.
The combination of pre-training and fine-tuning creates AI models that are versatile and powerful. They can be applied to a wide range of applications, from content creation to customer support and information retrieval. They've got it all covered.
What sets these models apart is their deep understanding of context. They produce text that rivals human-generated content, thanks to the knowledge acquired during their training. These models are the answer to the demand for natural language understanding and generation.
Of course, challenges exist when it comes to using pre-trained and fine-tuned models. The computational resources required can be a hurdle for many organizations, not to mention the time, cost, and effort in curating massive labeled datasets. And let's not forget the need to address biases in the generated text, as responsible AI is the way to go.
But there's more. Interpreting and explaining the behavior of these models is complex. With their size and complexity, uncovering the decision-making process behind their predictions is still an ongoing area of research. Transparent and ethical AI development is a priority, and efforts are being made to shed light on biases, errors, and ethical considerations.
Pre-trained and fine-tuned AI models have opened up endless possibilities in natural language processing and understanding. Their contextually relevant text generation and adaptability to specific tasks make them indispensable in various applications. As advancements continue, addressing challenges and promoting responsible and transparent AI development remain critical.
What is a Pre-trained Model?
These intelligent models have already undergone extensive training, allowing them to excel in specific tasks or applications.
During the pre-training phase, these models are exposed to vast amounts of unlabeled data, including text, images, and more. This process enables them to learn patterns, extract features, and understand the underlying structures and semantics of the input data.
But it doesn't stop there. After the pre-training phase, these models can be fine-tuned for specific tasks. By training them on labeled datasets that are tailored to the desired application, the models adapt their parameters to perform exceptionally well in real-world scenarios.
The beauty of pre-trained models lies in their ability to transfer knowledge. Their broad understanding of various domains, acquired during the pre-training phase, allows them to tackle specific tasks with ease, even with limited task-specific training data.
Not only are pre-trained models efficient, but they also save valuable time and resources. They eliminate the need to train models from scratch and deliver impressive results. From image recognition and natural language processing to recommendation systems, these models are revolutionizing AI research and applications.
In summary, pre-trained models are the key to unlocking AI's full potential. With their pre-training phase and fine-tuning capabilities, they offer a powerful and efficient solution for a wide range of tasks. Discover the power of pre-trained models and take your AI projects to new heights.
Enhancing NLP with Fine-Tuning: Boosting AI's Language Understanding
After a phase of pre-training that gives models a broad understanding of language, fine-tuning takes it to the next level, tailoring their knowledge to the intricacies of real-world languages.
During the fine-tuning process, the pre-trained model encounters a curated dataset specifically designed to enhance its performance on NLU tasks. By providing examples of inputs and corresponding outputs, this dataset equips the model with task-specific insights and guidance.
Fine-tuning refines the model's parameters, making adjustments to better align with the desired task. Through multiple training iterations, the model captures the subtleties of semantics, grammar, and context in natural language, resulting in more accurate predictions and coherent responses.
Prompt engineering is a key element in fine-tuning for NLU. By artfully crafting input prompts and instructions, developers can guide the model towards producing contextually appropriate and accurate outputs.
To further improve the fine-tuned model, human feedback is invaluable. Human evaluators assess the model's outputs, providing corrections and ranking them based on quality. The model learns from this feedback, aligning its performance with human-like language understanding.
Large-scale evaluation datasets have played a pivotal role in advancing the fine-tuning process. These datasets encompass a wide range of language phenomena, helping identify areas where the model may struggle and offering opportunities for targeted improvement.
The effectiveness of fine-tuned models stems from their sheer size and scale. With parameters and computational power like OpenAI's GPT-4 and Megatron-Turing NLG, these models capture complex language patterns and nuances. Their abundance of parameters enables them to learn from vast amounts of data and generate contextually appropriate and coherent responses.
Challenges accompany fine-tuning, demanding careful consideration of various factors. Overfitting, where the model becomes too specialized on the fine-tuning dataset, can be mitigated through regularisation and early stopping, ensuring robust performance across different inputs.
In a nutshell, fine-tuning is a vital component in improving AI systems' language understanding. By tailoring pre-trained models through labeled datasets, prompt engineering, and human feedback, these models excel at deciphering the subtleties of natural languages. With advancements in evaluation datasets and robust models, the potential for fine-tuning to enhance language understanding is boundless.
Statistical vs Machine Learning
The two main approaches to training language models are statistical and machine learning. By understanding their differences, you can unlock the potential for developing better models for natural language understanding (NLU).
Statistical approaches rely on probabilistic models that capture the statistical properties of languages. These models are efficient for applications like spell-checking or simple language generation tasks. However, they struggle with complex language phenomena and require manual crafting of linguistic features and rules.
On the other hand, machine learning approaches, particularly deep learning models, have gained attention for their remarkable performance in NLU tasks. These models learn directly from data, without the need for explicit feature engineering. They can handle long-range dependencies and encode more contextual information.
While machine learning approaches offer great potential, they also come with challenges. They require significant computational resources and training data, making them time-consuming and expensive. Fine-tuning and prompt engineering are crucial to achieve optimal results.
Understanding the trade-offs between statistical and machine learning approaches is key to designing and training language models for various NLU applications. Unleash the power of language models by making informed decisions.
Counting Parameters and Maximizing Efficiency
In 2023, the two key metrics that shape the capabilities and efficiency of large language models in AI are parameter count and FLOPS per parameter. These metrics hold the secret to creating models that can effectively capture complex language patterns.
Parameter count is the total number of learnable components in a language model. The more parameters a model has, the better it can grasp intricate linguistic nuances and excel in a range of natural language understanding tasks.
However, harnessing a high parameter count comes with challenges. Training and optimizing models with a large parameter count demand significant computational resources, making them time-consuming and costly to deploy.
On the other hand, FLOPS per parameter measures the computational efficiency of a language model. An optimized FLOPS per parameter ratio means the model can perform more calculations with fewer parameters, ensuring faster inference times and reducing the resources required for training and deployment.
Finding the right balance between parameter count and FLOPS per parameter is crucial. Striking this balance empowers researchers and practitioners to create scalable and practical language models that deliver both power and efficiency in various applications.
Don't overlook the importance of parameter count and FLOPS per parameter in the world of large language models. Achieve unparalleled understanding and generation of natural language by optimizing these critical metrics.
Decoding the Complexity of Large AI Models: Unveiling the Power of Parameter Counts
Parameter counts hold the key to unlocking the true potential of these models as they navigate and process information. Delve into the intriguing world of parameter count indicators and explore their ability to tackle the complexities of language patterns.
When it comes to language models, bigger is usually better. With larger models comes an increased parameter count, allowing for a greater ability to absorb and comprehend vast amounts of natural language data. Witness the remarkable prowess of these models as they master the intricate nuances and generate flawless natural language.
The parameter count takes center stage in the performance of language models. With an abundance of parameters, these models have the power to understand the subtle intricacies of linguistic patterns, thereby enhancing their performance in various natural language understanding tasks, including text completion, translation, and sentiment analysis.
But of course, with great power comes great challenges. Handling models with high parameter counts demands significant computational resources, from robust hardware to large-scale distributed training setups. The journey of training and deploying these models can be both time-consuming and costly.
Yet, researchers and developers are not alone in their quest. They rely on the metric of FLOPS per parameter, which measures the computational efficiency of a model. Combining this data with the parameter count unlocks valuable insights into a model's ability to perform computations with fewer parameters, optimizing efficiency to greater heights.
Enter the world of efficiency. Models with a higher FLOPS per parameter ratio redefine speed and practicality, offering real-time or near-real-time applications. Unleash the power of faster inference times for applications such as chatbots and virtual assistants. Experience the impact of reduced computational resources, making large AI models cost-effective and feasible even in resource-constrained environments.
Striking the perfect balance between parameter count and FLOPS per parameter is the true test for researchers and practitioners in the realm of language models. Their mission? To create models that flawlessly capture complex language patterns while optimizing computational efficiency. The delicate balance between these crucial factors shapes the landscape of large AI models, ready for deployment in a multitude of applications.
In conclusion, the complexity of large AI models unveils its secrets through parameter counts. These counts hold the key to their ability to understand and generate natural language flawlessly. As parameter counts rise, so do the challenges in terms of computational resources. Welcome the metric of FLOPS per parameter, a supreme insight into a model's computational efficiency. The delicate balance between these factors ensures the creation of large AI models that embody complexity and efficiency, destined to revolutionize numerous applications.
AI BLOG
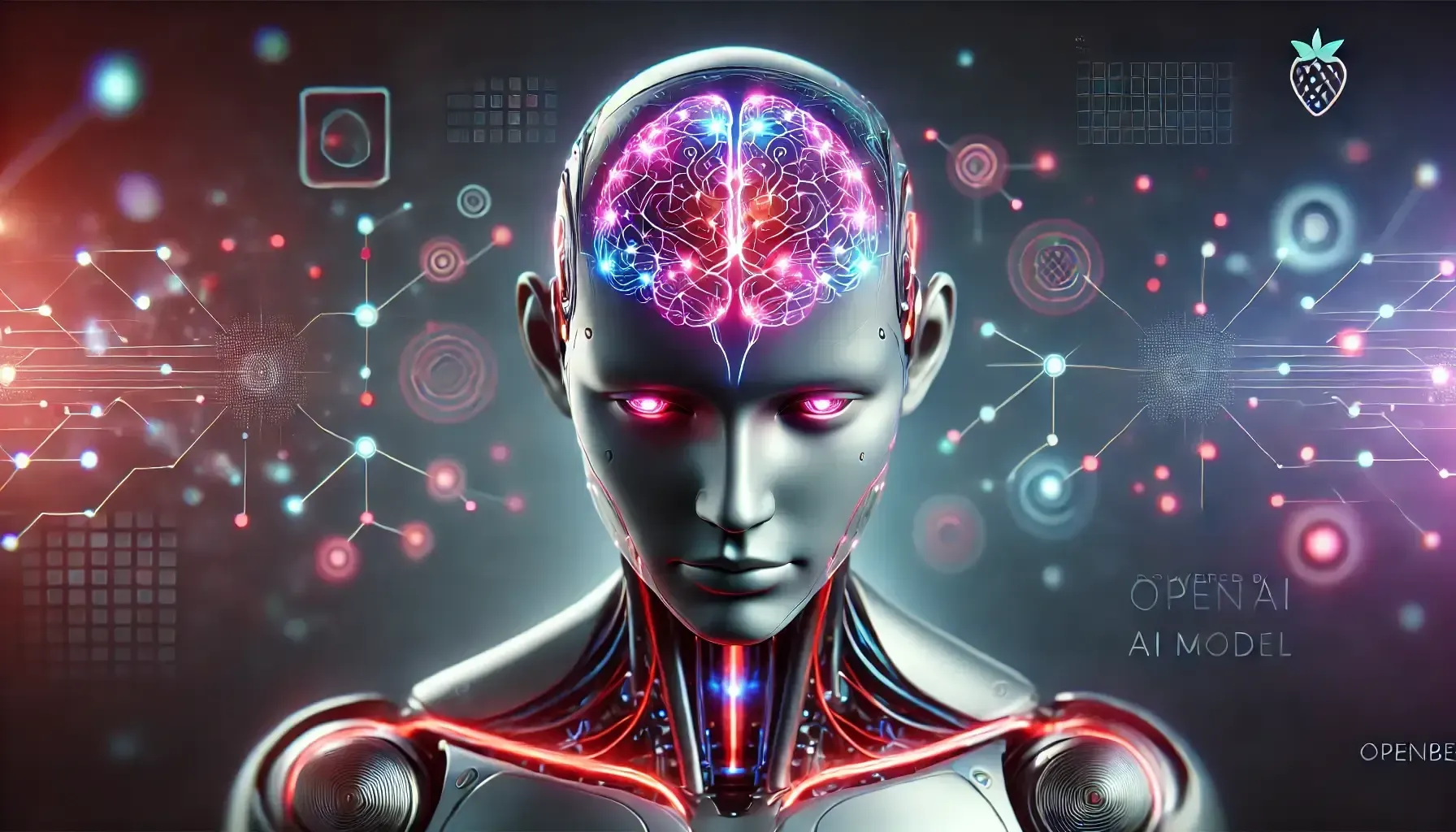
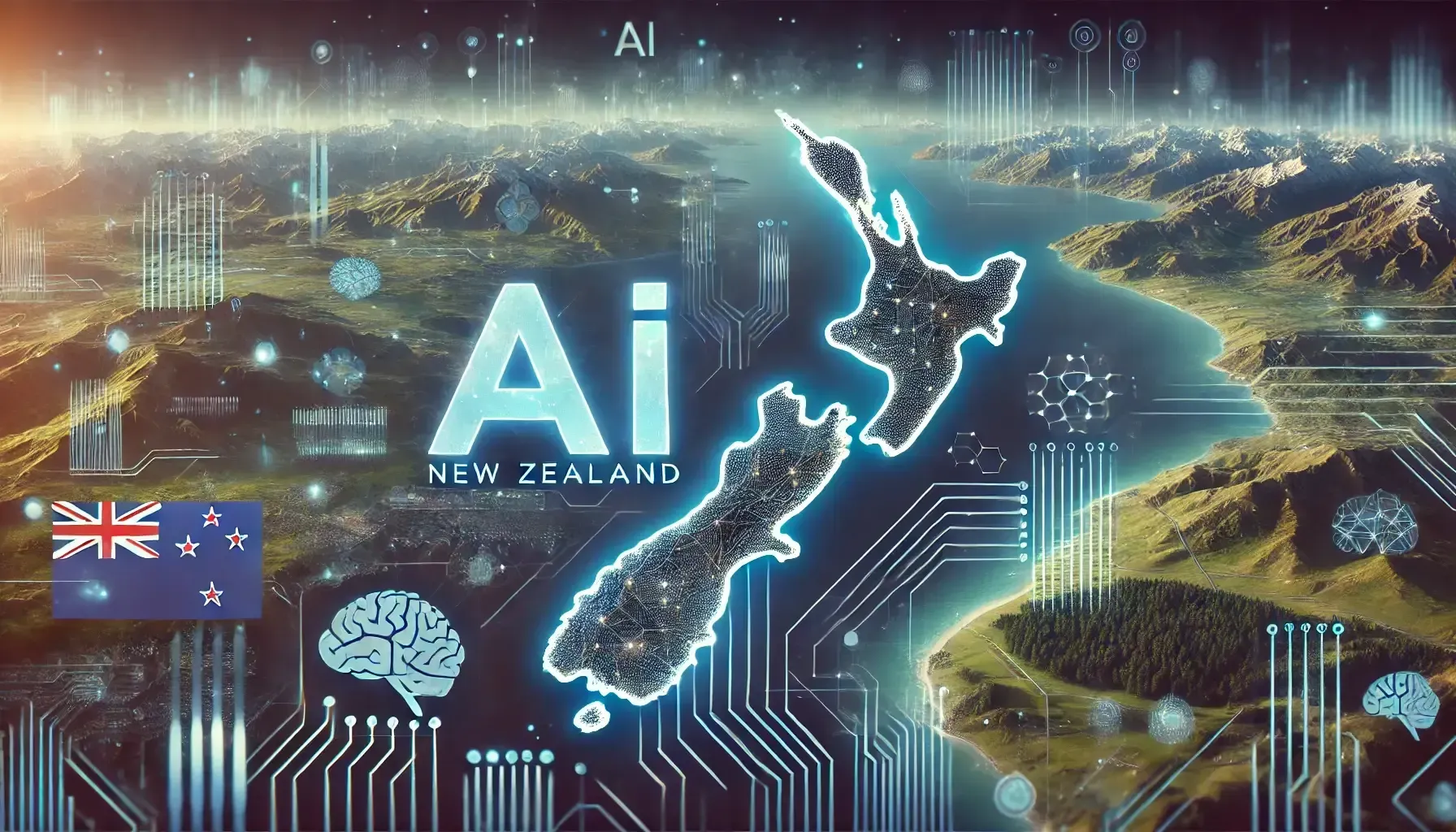
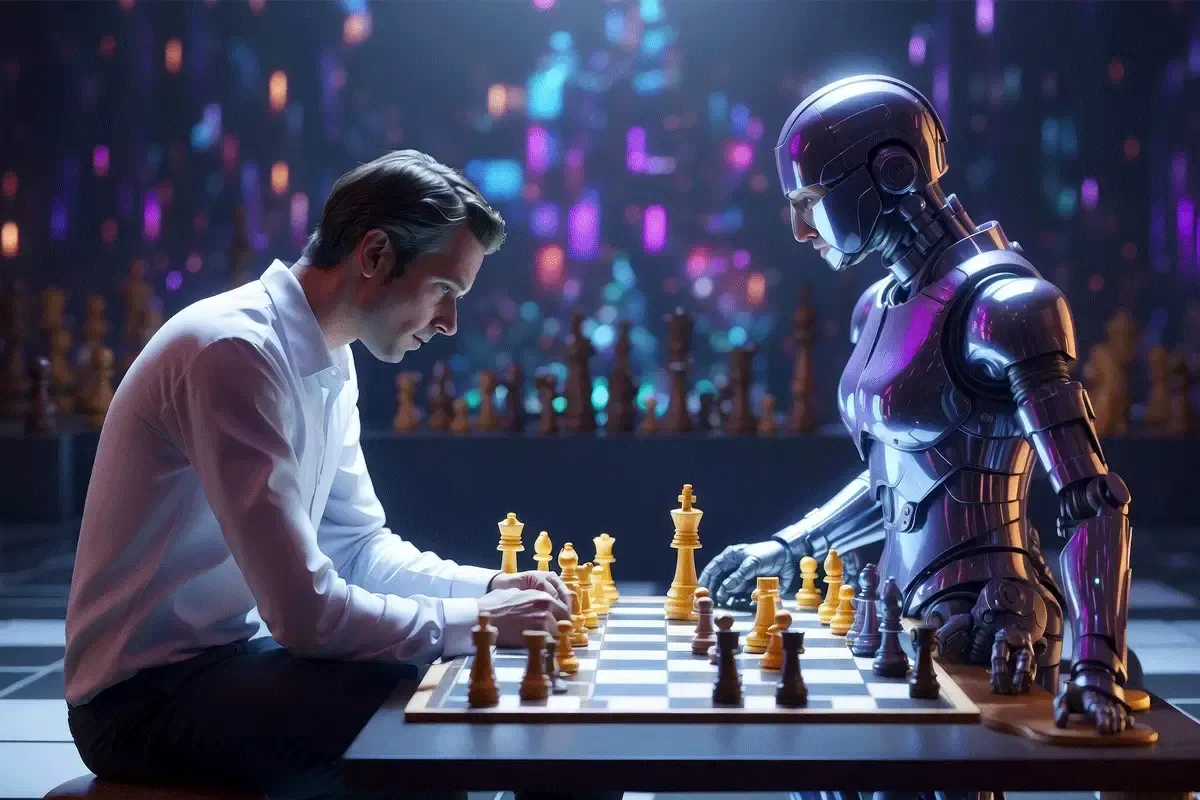